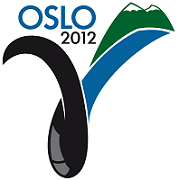 |
|
Ninth International Geostatistics Congress, Oslo, Norway
June 11 – 15, 2012 |
|
|
 |
|
No Paper Available |
Session: |
Theory 3 |
Abstract No.: |
O-071 |
Title: |
Revisiting the linear model of coregionalization |
Author(s): |
Denis Marcotte, Ã?cole Polytechnique, Department of Civil, Geological and Mining engineering (CA)
|
Abstract: |
The linear coregionalization model (LCM) is the model most commonly used in practice for cokriging. The LCM model amounts to represent the observed variables as linear combinations of sets of independent (or at least uncorrelated) underlying variables. The popularity of the LCM stems mostly from the ease of modelling and verification of the admissibility of the model. However, LCM has strong limitations as it implies symmetrical cross-covariances for the variables under study. The symmetry constraint could explain why the cokriging is generally unsuccessful to improve significantly over kriging when the observed variables are collocated. One possible generalization to the LCM (GLCM) is to consider the observed variables as linear combinations of underlying independent variables and of other dependant variables representing deterministic functions of some of the underlying variables. Interesting candidate functions are for example regularization, spatial shift and derivatives, the last two functions enabling to introduce asymmetry (and anisotropy) when needed. We note that GLCM comprises the deterministic cokriging of a variable and a given function of it (partial derivative, regularization,...) as a particular case. To ensure admissibility of the model, the coefficients of the underlying variables and functions are estimated directly using an iterative nonlinear regression approach that compares the theoretical covariances computed from the GLCM to the experimental ones (V-V plot). Candidate variables and functions are selected using a stepwise procedure so as to avoid over-parameterization of the model. Various synthetic cases are presented illustrating the flexibility of the GLCM. The well-known GSLIB U-V data file is also used to compare the relative performances of the LCM and of the GLCM. GLCM is shown to provide more precise estimations than the LCM model for the synthetic and test cases. It offers a more flexible and more general class of models than the LCM. |
|
|
|
|
|
Copyright 2012 International Geostatistics Congress
|
|